How IT executives leverage AI to predict the future and minimize surprise
Although the mountain of information can exhaust the limited time of a technology executive, a reasonable baseline understanding of the movements and cadence of artificial intelligence and predictive modeling is imperative to truly capture, and then, in turn, communicate, the enormous potential that some of these solutions hold.
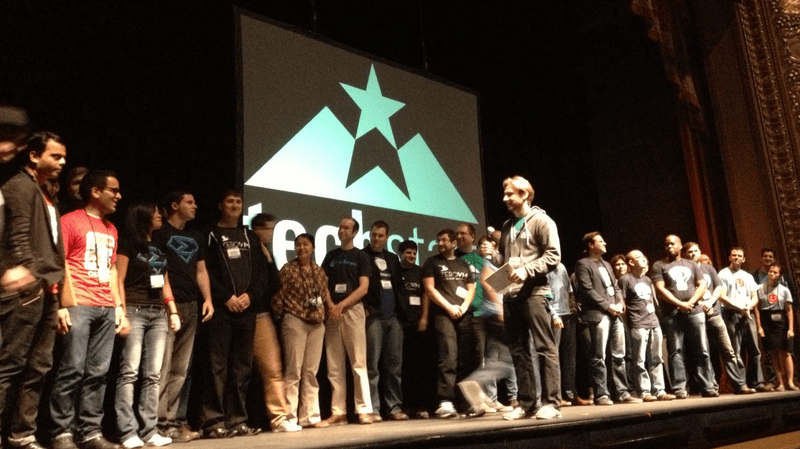
The teams pitched, the slides flew, the crowd lovingly cheered. But one company didn’t pitch. Jeremy, the co-founder and CEO, was nowhere to be found. Why? Because, apparently, he was on planes already, meeting privately with Venture Capitalists. DataRobot didn’t even need to pitch. The founder just took a few calls, and looked the VCs in the eyes and said something to the effect of, “I went to MIT, I can build models that will forecast pretty much anything, and we’re going to take this and run with it.” (Keep in mind, this is back in 2013, when we were all using iPhone version 5, and iOS 7. In 2013, the idea of predictive models were tied to excel spreadsheets and only math professors were really talking about it. There was no real artificial intelligence out there, no OpenAI or ChatGPT, and people just saw that is something out there, somewhere in the future.) That [oversimplified] pitch, was enough to raise millions in seed funding and set a powerful trajectory for DataRobot, now a unicorn and one of the darlings of the TechStars startup network.

DataRobot was founded in 2012 by Jeremy Achin and Tom de Godoy and is headquartered in Boston, Massachusetts. DataRobot has developed a platform that simplifies the process of developing machine learning models for businesses, regardless of their level of data science expertise. This platform streamlines the construction of models by automating crucial procedures like feature selection, model selection, hyperparameter tuning, and model interpretation. As a result, businesses can save time and resources while also ensuring the accuracy and reliability of their models. The DataRobot platform is utilized by a diverse range of industries, including banking, insurance, healthcare, and e-commerce. With the platform, businesses can develop models for various applications, such as predicting customer churn, detecting fraud, and creating product recommendation engines. It’s a great business to be in, and a powerful platform. They’ve built some truly amazing technology, and are way ahead.
Predictive models before AI
Early efforts to predict the future in a business sense focused on forecasting sales. This is an important part of budgeting. Mathematical models included weighted moving averages, exponential smoothing, and time series with trending and seasonality . As recently as 2022, Bosse and coworkers showed that in predicting COVID-19 cases and deaths, it was essentially a toss-up between experts and closed form mathematical models incorporating modern epidemiologic principles.
The balance has shifted. First came Cloud based relational databases chock full. The Human Genome Project required the shared infrastructure in order to have adequate data storage capacity. Data mining evolved into data analytics. This was facilitated by top down AI with machine learning. Computers using AI now are superior to Grand Masters in chess. There are a host of applications of this in business. One such application is predictive maintenance.
"Predictive maintenance allows us to move from a reactive mode, where we wait for something to break down before we fix it, to a proactive mode, where we can anticipate when maintenance will be needed and take action before a failure occurs. This approach can reduce downtime, improve equipment reliability, and lower maintenance costs." - Johnathan Foster, Director of IoT at SAS
Note in particular the references to reduced downtime and improved reliability. Surprises in the form of an unanticipated need to shut down a piece of equipment are costly beyond the maintenance itself. The desirable ability to minimize surprises is akin to a maximin strategy in game theory. Intelligent Manufacturing Systems (IMS) use tools such as Prognostics and Health Management (PHM) and concepts such as Maintenance Opportunity Window (MOW) to maximize availability and efficiency of machinery. PHM is a paradigm shift in which the condition of the asset is no longer essentially binary as it predicts the condition along a continuum of performance degradation, autonomously manages the situation, and optimizes product quality.
Lee and coworkers articulate a vision of the promised land, “The final aim is to allow the operation and all the assets within the operation to have self-assessment capabilities to evaluate their own performance and adjust based on process and operation parameters.”
Is today the tomorrow we predicted yesterday?
Although the great 6th Century Chinese philosopher Lao Tzu once said, “Those who have knowledge, don't predict. Those who predict, don't have knowledge,” I think we can all agree that our predictive powers have grown considerably since his time. If Lao Tzu had access to the technology and analytics software we have today, I’m sure he’d be revising his thinking.
In 2021, CTOs and CIOs have an abundance of options for utilizing their data. Real-time data capture and analysis require advanced AI capabilities. Business Intelligence tools like Tableau, Qlik, PowerBI, and Domo can help companies to manage their data silos and provide useful dashboards. These tools are useful for various applications such as CRM systems, HR, finance, and marketing datasets.
Cloud solutions are available for on-premises, private, public, and hybrid clouds. With these powerful tools, businesses can harness the power of data and utilize it effectively for forecasting and analysis. The ability to capture and analyze data in real-time is a crucial aspect of modern business.
Data integration tools are also important components of data management. They can help clean, prep, and transform data on the fly. These tools are necessary for accurate and reliable forecasting purposes. Companies must have the capability to process vast amounts of data quickly and efficiently.
Overall, the importance of data management, real-time data capture and analysis, and forecasting cannot be overstated. With the right tools and strategies, CTOs and CIOs can make informed decisions and drive business success. Predictive analytics are used in a number of areas, including:
- Financial forecasting
Data and analytics can be used to analyze financial data and trends, such as stock prices and interest rates, to make informed projections about future financial outcomes. - Supply chain management
By analyzing data on supply chain performance, businesses can identify areas for improvement and make projections about future supply chain operations. - Sales forecasting
Data and analytics can be used to predict future sales trends, based on historical data and market trends. - Marketing analytics
By analyzing data on customer behavior and marketing performance, businesses can make projections about future marketing campaigns and identify areas for optimization. - Human resources forecasting
By analyzing data on employee performance and turnover rates, businesses can make projections about future workforce needs and develop strategies for recruitment and retention. - Risk management
Data and analytics can be used to identify potential risks and make projections about future risk scenarios, allowing businesses to develop proactive risk management strategies. - Operational forecasting
Data and analytics can be used to optimize operational processes and make projections about future operational efficiencies. - Predictive maintenance
By analyzing data on equipment performance, businesses can make projections about future maintenance needs and develop proactive maintenance strategies to minimize downtime and costs. - Customer analytics
By analyzing customer data, businesses can make projections about future customer behavior and develop strategies to improve customer satisfaction and retention. - Energy forecasting
Data and analytics can be used to predict energy demand and make projections about future energy usage, allowing businesses to optimize energy consumption and reduce costs.
Actual companies that have applied predictive analytics successfully
American Express
American Express uses predictive analytics extensively to identify and prevent credit card fraud. They use advanced machine learning algorithms to analyze millions of transactions and identify patterns of fraudulent activity.
The company has a proprietary system called the "Proactive Risk Identification and Control" (PRIC) system that uses predictive analytics to identify fraudulent transactions in real-time. The PRIC system uses several techniques, including behavioral analysis, to identify suspicious patterns of activity and flag potential fraud.
American Express also uses predictive analytics to monitor customer behavior and identify potential fraud risks. They analyze data such as customer demographics, transaction history, and spending patterns to identify anomalies and detect fraudulent activity.
Overall, American Express's use of predictive analytics has been a key factor in their success in the credit card industry. By using data and analytics to identify potential fraud risks and personalize the customer experience, the company has been able to improve customer satisfaction, reduce losses, and increase profitability.
Proctor & Gamble
Procter & Gamble (P&G) has implemented predictive analytics to optimize its supply chain operations and forecast the demand for its products. Advanced analytics and machine learning algorithms allow P&G to predict consumer demand accurately, manage inventory levels efficiently, and improve production planning.
P&G collects massive amounts of data on consumer behavior, market trends, and production processes. It analyzes this data to develop precise demand forecasts. Predictive analytics enables P&G to anticipate changes in demand and make adjustments accordingly. As a result, the company can reduce waste and increase efficiency.
In addition to demand forecasting, P&G also uses predictive analytics to optimize its supply chain operations. By analyzing data on transportation routes, inventory levels, and production schedules, P&G can identify areas for improvement and increased efficiency. AI powered advanced analytics translate anticipated demand into actionable output even for complex upsteam supply chain configurations. Humans commonly produce errors that are progressively magnified. The result is the IT equivalent of the Galloping Gertie: the so-called bullwhip effect. AI driven analytics are clearly superior to humans in this application. P&G has developed a tool called the "Virtual Control Tower," which utilizes predictive analytics to monitor and manage the entire supply chain in real-time.
When minimizing surprises is actually achieved
No one likes bad news, anticipated or not. If you call that a surprise, just FYI that is unexpected bad news. Surprises are fun, like surprise parties, surprise gifts, and an unexpectedly high bonus at work. Companies that give big bonuses are able to do so because they dislike surprises. Running a company is like piloting a commercial airliner. The paradigm is the Conservative Response Rule, which states that anytime a pilot is faced with a choice or decision, they make the conservative one. The one that minimizes risk to the revenue stakeholders. They are wise enough to deviate to the alternate airport and bear the brunt of passenger annoyance rather than accept any increased risk of a disaster.
Those who lead large publicly traded corporations often face a difficult balancing act. The basic principle of investment and finance is that risk and potential reward are directly related. While this may not be intuitive, it is undoubtedly true. Therefore, wise corporate leaders are willing to reduce potential rewards to limit risk. After all, corporations can crash just as surely as an airliner.
When it comes to AI-driven data analytic applications, companies must provide guidance beyond simply maximizing the bottom line. Focusing on profit alone may lead to results analogous to attempting to set the low altitude record in an airplane. Shooting for the stars is fine, but there is a risk of becoming a shooting star...
There is wisdom in running a long race like the tortoise rather than the hare. Companies aspire to be successful for the long term. Corporate leaders- including AI driven data analytic applications that make predictions- must balance the desire for growth and profitability with the need to mitigate risks and ensure sustainability. By doing so, they can achieve lasting success and avoid the pitfalls that can come with taking excessive risks.
Minimization of potential losses is understood in this context as inherent in good stewardship of stakeholder investment. Game theory has been driven primarily by equilibrium based models since John Nash’s work in the 1950s. The minimax approach gives priority to choosing the strategy with the best worst scenario. As it is a one-sided strategy for competition with a hostile opponent in a noncooperative context, it is well suited for the dog eat dog realities of competition in the market place. Sometimes it is best to ensure one lives to fight another day...
Where we are and where we are going with predictive analytics
Data quality tools use AI and machine learning to automate the data quality process, which helps users generate more accurate results while also saving considerable time. Gartner expects the data quality vendors to add data integration, metadata management, and Master Data Management functionality in the coming quarters, making these tools lesPhases Involved in Providing Quality Informations niche that should help broaden their appeal.
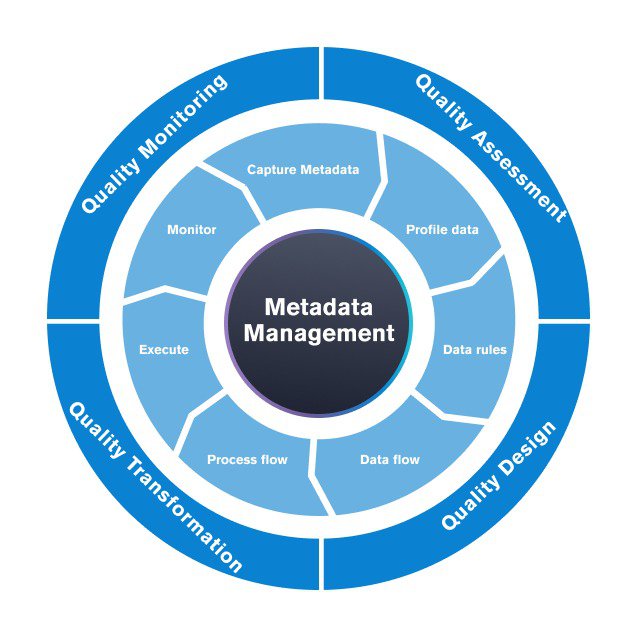
According to Gartner, an ABI platform should contain the 15 critical capability areas, including such unassuming things as security, manageability, access to cloud platforms, connectivity, data prep, the ability to build complex models, as well as more sophisticated processes like creating advanced analytical models, providing natural language query and natural language generation (NLG), and, of course, producing pixel-perfect reports. Above all else, today’s ABI platforms should be able to tell a story with data, combining interactive visualization techniques with a narrative that can deliver insights in compelling and easily understandable ways for both the executive suite and, in some cases, the frontline workers. We’ve come a long way since the days of generating multi-colored pie charts and bar graphs in Excel. We can now build sophisticated mashups of real-time streaming data with full drill-down functionality on data run sophisticated predictive and prescriptive models.
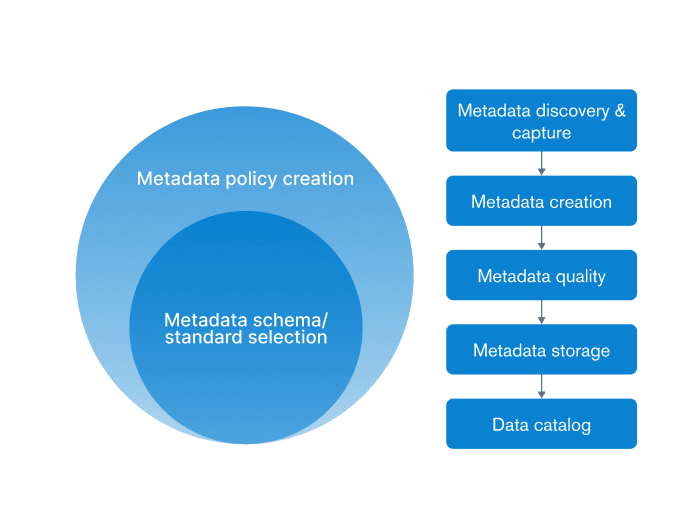
When it comes to analytics, Gartner sees “a healthy market that is maturing rapidly.” In its Magic Quadrant for Data Science and Machine Learning Platforms. They define a DSML platform that helps with data ingestion, data prep and exploration, model creation, training, testing, and deployment. While expert data scientists might choose to code their data science models, there’s a movement towards drag-and-drop UIs that create visual pipelines for an emerging class of analysts known as ‘citizen data scientists’.
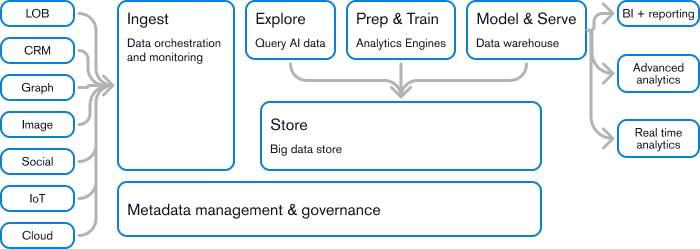
Mark Twain once said, “it is difficult to make predictions, particularly about the future.” And that’s doubly true when it comes to IT. A hundred years ago, who would have thought in 2020 you could fly 40,000 feet in the air while talking to friends on a mobile phone over a social channel that can help you connect with over a billion people? Today – and always, business has been a literal bet on a prediction or the future; a billion-dollar casino doesn’t rise in deserts of Nevada if the banks and shareholders putting up the money don’t see it as a great investment; an e-tailer doesn’t fill its warehouses with products it believes are going to sit on the shelf collecting dust; a factory doesn’t hire workers if it can’t keep them busy making a sellable product; a restaurant doesn’t cook food if it thinks it will all go into the trash at the end of the night. So, it is with technology, which is rare in that it can get gamblers into that casino, or help products fly off that e-tailer’s shelves or sell food for an in-house diner or a delivery customer.
The author and futurist Karl Schroeder once said, “Foresight is not about predicting the future, it's about minimizing surprise.” In these most surprising of times, it’s a sentiment most CIOs and CTOs can surely get behind.
Takeaways
- Focusing on the quality of data is imperative to establishing your predictive modeling metrics. Garbage-in, garbage-out has never been more true. The accuracy and completeness of the data used in predictive analytics is critical to achieving accurate results and minimizing surprises.
- Developing effective models that accurately represent the relationships between variables is essential.
- Predictive models should be continually refined and updated based on new data and changing circumstances to ensure they are in step with the business and the IT leadership, as well as the overall executive team.
- Directly and deeply integrating predictive analytics into existing business processes can help to go even further down the automated, predictive-driven pseudo-neural network approach, where data drives the actual steering of the ship. This is scary to some, but is indicative of the future.
- Scenario planning can be done upfront. The leadership and steering committee(s) that drive the predictive modeling group can foster, encourage, steer, and develop multiple scenarios based on different assumptions.
References:
Bosse, N. I., Abbott, S., Bracher, J., Hain, H., Quilty, B. J., Jit, M., ... & Funk, S. (2022). Comparing human and model-based forecasts of COVID-19 in Germany and Poland. PLoS computational biology, 18(9), e1010405.
Lee, J., Ni, J., Singh, J., Jiang, B., Azamfar, M., & Feng, J. (2020). Intelligent maintenance systems and predictive manufacturing. Journal of Manufacturing Science and Engineering, 142(11).
Schwecke, T. J. Applications of Predictive Analytics: Evolution, Integration in Modern Society, and Ethical Concerns. https://uwm.edu/actuarial-science/wp-content/uploads/sites/549/2021/06/annotated-Predictive_Analytics_Paper-7.pdf
The room was packed Demo Day for technology accelerator, TechStars, most recent class of startups. It was May of 2013, and 12 groups of bright, young, and talented founders were scuttled to the backstage of an old theatre in San Antonio, Texas. Demo Day was a chance for the founder CEOs to stand on stage and pitch their tech startups to investors, family, and friends. Getting to this point required months of rework, rehearsal, founder scuffles, code, and even pivots. Every PowerPoint slide was loaded and ready, complete with each picking their own stage walk-on music. Among the batch of founders was the core group of a little known startup called DataRobot.